What You Need to Know About Analog Computing
December 07, 2021
Story
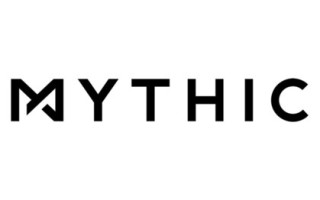
As artificial intelligence (AI) and deep learning applications become more prevalent in a growing number of industries, the need for better performance, larger deep neural network (DNN) model capacity, and lower power consumption is becoming increasingly important.
At the same time, DNN models are growing at an exponential rate. With these models, traditional digital processors struggle to deliver the necessary performance with low enough power consumption and adequate memory resources, especially for large models running at the edge. This is where analog computing comes in, enabling companies to get more performance at lower power consumption in a small form-factor that’s also cost-efficient.
Analog compute has been researched for decades, and provides two main benefits. First, it is amazingly efficient; by leveraging the memory element for both neural network weight storage and computation, it eliminates data movement. Second, it is high performance and low-latency, making it suitable to compute the hundreds of thousands of multiply-accumulate operations occurring in parallel during vector operations. Given these two factors, analog computing is ideal for the latest edge-AI computing requirements.
The computational speeds and power efficiency of analog compared to digital has been promising for a long time. But even beyond the incredibly difficulty of developing this technology, one of the biggest historical impediments of analog compute has been its size, with analog chips and systems being far too big and costly. Today, combining flash memory and analog compute solves these challenges, and you get a sum that is far greater than the individual parts – analog compute-in-memory. This is the unique technology that Mythic has spent years perfecting and has now demonstrated, and it sets the stage for AI compute for decades to come.
The power advantages of analog compute-in-memory (or analog compute) at the lowest level comes from being able to perform massively parallel vector-matrix multiplications with parameters stored inside flash memory arrays. Tiny electrical currents are steered through a flash memory array that stores reprogrammable neural network weights, and the result is captured through analog-to-digital converters (ADCs).
By leveraging analog compute for the vast majority of inference operations, the analog-to-digital and digital-to-analog energy overhead can be maintained as a small portion of the overall power budget and a large drop in compute power can be achieved. There are also many second-order system level effects that deliver a large drop in power; for example, when the amount of data movement on the chip is multiple orders of magnitude lower, the system clock speed can be kept up to 10x lower than competing systems and the design of the control processor is much simpler.
Using analog compute processors for edge-AI applications is a great option for many different use cases. For example, drones equipped with high definition cameras for computer vision (CV) applications that require running complex DNN models locally to provide immediate and relevant information to the control station. Processors that use analog compute make it possible to deliver powerful AI processing that’s also extremely power-efficient so companies can deploy these networks on the drone for a wide range of CV applications. These applications include monitoring agricultural yields, inspecting critical infrastructure such as power lines, cell phone towers, bridges and wind farms, inspecting fire damage, and examining coastline erosion. Another type of application that analog compute is ideal for is low-latency human pose estimation, which can be used in smart fitness, gaming, and collaborative robotics devices.
Analog compute is the ideal approach for AI processing because of its ability to operate using much less power at a higher speed with faster frame-rates. The extreme power efficiency of analog compute technology will let product designers unlock incredibly powerful new features in small edge devices, and will help reduce costs and a significant amount of wasted energy in enterprise AI applications. Leveraging analog computing power combined with flash memory, OEMs can rethink what is possible with AI. Just imagine what exciting innovations we will see without the existing limitations on edge-AI applications’ power, cost, and performance.