CEA-Leti Scientists Demonstrate Machine Learning Technique Exploiting RRAM Devices
January 19, 2021
News
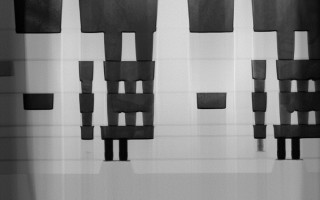
CEA-Leti announced that its scientists have demonstrated a machine-learning technique exploiting what used to be considered non-ideal traits of RRAM devices. This overcomes barriers in the development of RRAM-based edge-learning systems.
CEA-Leti announced that its scientists have demonstrated a machine-learning technique exploiting what used to be considered non-ideal traits of RRAM devices. This overcomes barriers in the development of RRAM-based edge-learning systems.
In the January edition of “Nature Electronics,” the research team at CEA-Leti portrayed how RRAM, or memristor technology is able to be used to create intelligent systems that learn locally at the edge. The algorithm, which is sued in current RRAM-based edge approaches, can’t be reconciled with device programming randomness or variability.
In order to subside the problem, the team developed a method that actively exploits that memristor randomness, implementing a Markov Chain Monte Carlo (MCMC) sampling learning algorithm in a fabricated chip that acts as a Bayesian machine-learning model.
According to a company press release, while machine learning provides the enabling models and algorithms for edge-learning systems, increased attention concerning how these algorithms map onto hardware is required to bring machine learning to the edge. Machine-learning models are normally trained using general purpose hardware based on a von Neumann architecture, which is unsuited for edge learning because of the energy required to continuously move information between separated processing and memory centers on-chip.
RRAM technology has been applied to in-memory implementations of backpropagation algorithms to implement in-situ learning on edge systems.
For more information, visit http://www.leti-cea.com/.