Industrial AI: Predictive Maintenance on Steroids
October 24, 2023
Blog
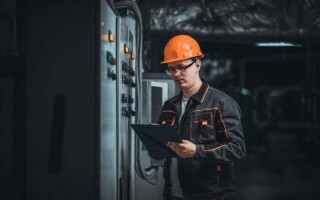
Since the Industrial Revolution, asset-intensive organizations engaged in a systematic "pen and paper" approach to inspect, evaluate, and diagnose equipment, machinery, and infrastructure to identify potential issues, assess severity, and recommend appropriate actions.
Inspectors walked along assembly lines to conduct visual inspections of output, drivers checked vehicles for functionality, workers checked individual components of tools, and operators regularly checked machinery to confirm the appropriate operation.
Manual inspections are slow and require the inspector to have decades of experience in order to accurately identify problems. As more and more of these experienced subject matter experts retire, and a shrinking number of new entrants backfilling them, this approach was not sustainable.
In 2006, the term smart manufacturing was coined to represent technology-driven approaches that utilized large-scale optimization, sensor networks, data interoperability, and requirements-driven security. The incorporation of the Internet of Things (IoT) drove the transformation with networks of interconnected devices, sensors, and software that monitored and optimized the production process. Smart assets evolved into collecting and sharing data while providing proactive feedback on that asset's economic, environmental, health, and safety performance in aggregation with the other connected assets.
The advent of IoT created an explosion of information like none other in history. According to an International Data Corporation report, 175 zettabytes (ZB) of data are anticipated to be generated by 2025. Of that, approximately 80 ZB of data is attributable to IoT devices.
Asset-intensive companies are now turning to artificial intelligence (AI) to harness the power of the data. AI facilitates increased reliability and the availability of equipment while curbing maintenance costs. By coupling IoT with advanced analytics capabilities alongside traditional lean tools and techniques, equipment failures can be predicted and prevented while leaving assets that do not need maintenance to continue running smoothly.
Predictive Maintenance
Predictive maintenance uses machine learning algorithms to ingest historical data from sensors and maintenance logs to identify patterns in the data. When provided with sufficient information, the collected data can be used to build a model that outlines normal operations. The normal behavior model can then analyze facility data in real time and identify and flag any values that deviate from the established norm. When something outside normal operations is identified, the model can pinpoint precisely when and how a failure will occur – a proactive approach to asset maintenance and management. Just like that expert with decades of experience, the model can call on the thousands of years of combined experience stored in decades of maintenance logs.
Machine learning also maintains the models over time. With traditional predictive models that don't employ AI, a change in even a single variable, such as a replaced part, necessitates reworking the entire model. This also applies to the normal changes an asset goes through over its life span – i.e., a component that has been in service for an extended period does not perform the same as when it is brand new. Machine learning (ML) models dynamically learn and maintain themselves by adjusting to any component or asset and adapting to changes. This means that as the model is exposed to more variability in asset performance, it becomes more resilient to natural changes from asset use.
When systems or subsystems do not provide the type or amounts of data predictive maintenance requires, SparkCognition's Deep ML-powered natural language processing (NLP) technology can decipher and use unstructured data, including PDFs, books, journals, audio, video, images, notes, analog data, and a range of other sources. With NLP, predictive maintenance models can use data sources beyond sensors, including all asset-associated data, such as maintenance records. By extracting facts, figures, entities, and contextual data from an asset's maintenance history, predictive maintenance solutions outfitted with NLP find causal patterns that indicate potential failures, even in so-called dark subsystems that lack sensors.
NLP technology takes asset management one step further by ingesting historical records, service manuals, and past courses of action by subject matter experts to speed up maintenance processes by listing possible next steps and suggesting corrective measures.
The result, artificial intelligence (AI) compounds the benefits of assets and connected devices by interpreting conditions and making decisions without requiring direct human intervention. The human-like awareness and decision-making increase efficiency and improve processes. It's predictive maintenance on steroids.
Milton Lopez is an accomplished design leader and innovator with extensive experience in UI development for AI solutions. He serves as the Head of Design at SparkCognition, leading a team of designers to create cutting-edge user experiences. Milton is also the Director of Applied Solutions at SparkCognition, where he oversees innovation projects combining physical and virtual systems to demonstrate the impact AI can have in the real world.
With a Bachelor of Arts degree in Liberal Arts and Sciences from the University of North Texas, Milton's multidisciplinary background enables him to approach design challenges from a holistic perspective. He is dedicated to delivering exceptional solutions that meet the needs of the users and exceed their expectations.