How Multimodal AI Will Shape the Edge
May 05, 2023
Blog
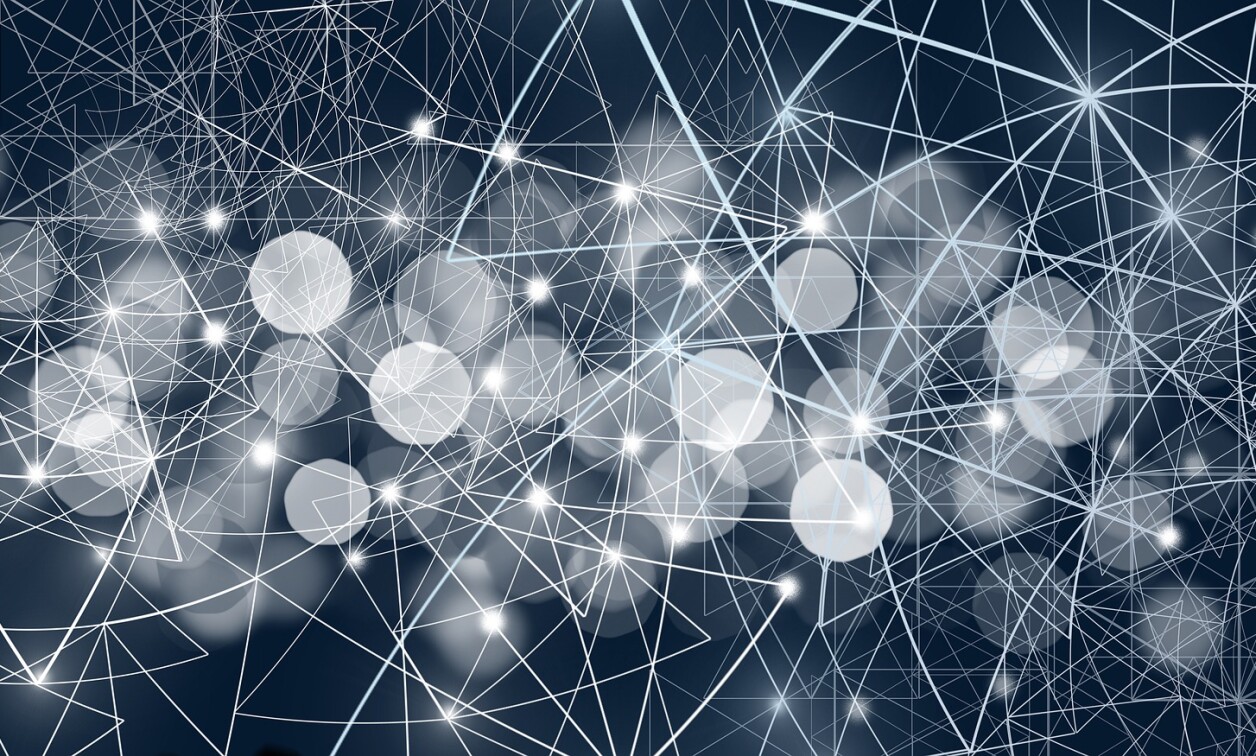
The recipe for designing and deploying IoT devices and services has been written and is now undergoing substantial refinement. Advances in sensor technologies, device and data security, and device interoperability are making it easier to collect valuable device and user data than ever before. With exponential growth in IoT data, methods to process and deliver critical insights are struggling to keep pace, especially where systems cannot rely on the cloud.
For these reasons, enabling devices to analyze sensor data and make real-time decisions using embedded machine-learning approaches is becoming a major focus. However, traditional processor architectures are very limited in supporting multi-sensor systems leading to neural processor architectures and software structures as the future of on-device AI.
We are still in the early evolutionary stages of embedded neural processors, with many taking a narrow use-case-defined approach. Future edge processors need to be capable of processing multiple sensor data types concurrently to achieve the full benefits of on-device AI. The path to delivering such solutions within highly constrained size, weight and power conditions is riddled with snags and diversions.
The rise of IoT from the home to the manufacturing floor has ushered in a period of disruptive technologies redefining products across every industry. Driven to obtain customer loyalty in an intensely competitive market. Product manufacturers embracing IoT technologies strive to deliver a brand-identifying customer experience. One key to acquiring brand loyalty is understanding more about the user than the user commonly understands themself.
Device and equipment manufacturers must balance valuable customer and device data collection without jeopardizing customer trust. Acquiring such data as how, when, where, and how often the manufacturer’s product is used typically involves sensors to measure every aspect of operation. Equally, devices commonly include wired or wireless connectivity to collect this data and a user app enabling customization or enhanced services based on user preferences. Manufacturers can broaden the wealth of data by expanding sensors and connectivity across their product portfolio, enabling data-driven decisions on future product specifications, and thereby continuing the innovation cycle.
As product designers continue to invent new ways to gain valuable insights from ordinary physical objects, sensors are rapidly expanding in scope. As costs decrease, manufacturers integrate more sensors resulting in more data generated per device. As this trend permeates across industries, device data expands exponentially.
Despite the competitive pressure, many companies struggle with the fundamental skills and infrastructure to analyze this multiplicity of data, assuming they have solved the data capture problem. Meanwhile, those accelerating their competitive advantage are doing so through the incremental process, operation, and supply chain improvements enabled by IoT data. However, even the most sophisticated are becoming overwhelmed by the volume of data outpacing the network, storage, compute, and energy capacity required to stay ahead. Two reports suggest as much as 73% to 97% (Forrester and Gartner, respectively) of data sits unused for analytics.
While commonly considered a topic of science fiction stories, hyperscale cloud services have been leveraging artificial intelligence (AI) to service industries' data-crunching demand for over a decade. However, the recent hype built from the accessibility of apps like ChatGPT and DALL-E has moved AI from geek speak to supermarket conversations. These Large Language Models (LLMs) are a testament to the seemingly endless potential of Generative AI.
LLMs are also prime examples of the exponential rise in cost and energy consumption required to produce each model, which is exponentially more complex than its predecessor. Training GPT-3, the root of ChatGPT, is estimated to consume over 30 days and a staggering $4.6M. While exorbitant training costs attributed to LLMs receive considerable attention, inference applications, such as ChatGPT, consume far more resources due to near or constant run rates. Given the resource constraints of edge devices, one can ask if LLMs could be enabled in anything other than the cloud.
History has answered such questions by regularly demonstrating that which can run in the cloud eventually runs on mobile and remote devices. If cloud computing may define the past decade, the next will be distributed AI applications across the many network layers from the cloud down to constrained end-point devices and sensors.
This continuum is the edge, where numerous demands create a diverse and distributed multiclass system of systems. Here, real-time data processing is unserviceable because of the long transmission delays of centralized cloud-based services. Increasing consumer concerns such as data privacy and security, intensify the pressure to move AI algorithms to the source of the data.
(Source: Semiconductor Engineering)
However, achieving this outcome requires advancing AI hardware and model optimization techniques to efficiently process data generated from the many sensors on an edge device. Current AI hardware architectures typically run a single model at a time, with some limited to a narrow range of sensor and model types, also called modes. Rapid innovation in neural processors, a relatively new class of processor architecture, is broadening the spectrum of efficient, on-device AI. As a result, SoC architects are tackling a new level of hardware and software integration complexity while maintaining strict size, power, and cost constraints.
To address demand, emerging edge solutions supporting diverse sensor types, often called multimodal, are gaining momentum. At the chip level, multimodal solutions commonly integrate a heterogeneous system of processor flavors tuned for the varying sensors in a single system-on-chip (SoC). Providing each processing element with sufficient memory and communication bandwidth requires an astute understanding of use case dependencies to avoid contention and failed execution.
For example, a surveillance solution's bandwidth requirement to process 4K video at 60 frames-per-second is far different than a remote health monitor tracking patient vitals and activity. Combining each processor's SW tuned to its use case introduces another complexity level with each added processor, all managed by a central host CPU. Such approaches are not new, but the chip size, power consumption, and hardware and software integration complexity present extreme practical limits closest to the sensor.
In parallel, multimodal AI models are trained with multiple data types, such as visual, textual, and audio, to construct a broader contextual understanding like human cognition. In these applications, a device would see, hear, and even feel its environmental conditions to recognize objects, describe their observed state, and even predict future outcomes. The recent launch of GPT-4 and research such as Meta's Project CAIRaoke illustrates advances in digital assistants to become conversational and intuitive. Like other generative AI predecessors, multimodal models are highly complex, consume significant hardware resources, and are difficult to deploy at the edge. Despite notable progress, numerous challenges remain, and by their own admission, Meta's goal of natively running such models on edge devices like AR/VR glasses is years away.
Given edge devices will always be constrained, new hardware and AI model approaches are needed to meet the concurrent multimodal applications with expanding demand in industries ranging from automotive to the Metaverse. Edge AI models will be handcrafted or distilled from foundation models and optimized for specific use cases.
On-device AI accelerators will need the flexibility to support different sensor modes while simultaneously processing multiple models to deliver accurate real-time results. Autonomous delivery robots must simultaneously recognize objects while calculating location and navigation to reach its destination and virtual reality glasses must concurrently track eye gaze while processing multiple video and audio inputs to deliver a realistic user experience.
Leveraging fewer processing elements will simplify system-on-chip integration, accelerate hardware and software development, and reduce the cost of deployment. We have entered the age of edge computing, where any device class, from the sensor to the cloud, will be enabled to address the ever-expanding realm of AI use cases across a distributed intelligent edge.
Conclusion
After more than a decade of moving data to the cloud, real-time edge requirements, data privacy, and security concerns are forcing us to rethink pushing artificial intelligence to the data. On-device data analytics is becoming crucial across every industry, yet the expanding number of sensors per device generates volumes of data unserviceable by contemporary processor architectures.
In parallel, AI algorithms continually evolve, with recent examples like ChatGPT, DALL-E, and GPT-4 expanding capabilities by leaps and bounds. Moving these disruptive technologies to the edge is limited by device constraints, such as cost, size, weight, and power. While novel neural processor architectures are expanding possibilities, innovative optimization techniques are needed to lower the barrier to adoption.
Recently, researchers ran a version of the popular image generator, Stable Diffusion, on an Android phone, creating images in under 15 seconds. Given the compute-intensive nature of the model and the constraints of the device, this impressive achievement symbolizes the future of on-device AI.
As these complex models are distilled and optimized for edge use cases, edge device and equipment manufacturers must accommodate multiple compact models, often running concurrently, to deliver competitive advantage and coveted customer loyalty.