JUST: An End-to-End Approach for Multilingual ASR
March 03, 2022
Blog
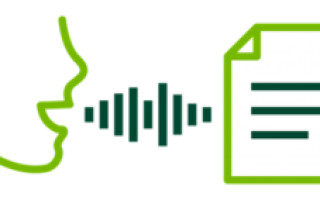
There is an increasing demand for the use of automatic speech recognition in customer service agencies and corporations to reduce procedural complexity. To bring competitive performance and architectural simplicity, an end-to-end automatic speech recognition system has emerged as a savior.
Within the ongoing research on improving performance and accuracy associated with the ASR, self-supervised training has grown as an effective methodology for pre-training models and downstream finetuning. In the initial days of the innovation, the research delves into a 2-step training stage where self-supervised loss is optimized in the first pre-training state.
However, these methods only contain one self-supervised loss in the optimization. In the proposed methodology for multilingual ASR through an end-to-end Joint Unsupervised and Supervised Training (JUST), the goal is to incorporate two self-supervised losses — contrastive and masked language modeling (MLM) losses. The problem with finetuning a pretrained model is the possibility of catastrophic forgetting.
With a large dataset, the model tends to forget the previously exposed knowledge when trained under supervision. Also, the issues related to the pre-trained checkpoint selection are more serious in multilingual ASR as different languages are heterogenous. The evaluation was done using an open-source dataset Multilingual LibriSpeech that consisted of 8 languages and was highly unbalanced.
The JUST framework consists of several modules that take care of the unsupervised and supervised losses. As shown in the overview of the JUST framework, all the modules (except Quantizer) take the output from the previous modules, which are stacked sequentially. Along with this, the unsupervised target vectors and IDs are for contrastive and MLM losses respectively, while the supervised targets are for RNN-T loss. All the modules with their respective losses are explained in the research paper.
[Image Credit: Research Paper]
The open-source dataset employed has 8 languages: English, German, Dutch, French, Spanish, Italian, Portuguese, and Polish with over 44 hours of English and 6 hours of the other languages combined. Polish is a low-resource language that only has 100 hours. But JUST framework shows the capability of handling low-resource languages in multilingual ASR. In the evaluation of the framework, the team explores JUST trained from scratch and finetuned from a pre-trained checkpoint.
“Average work error rate (WER) of all languages outperforms average monolingual baseline by 33.3%, and the state-of-the-art 2-stage XLSR by 32%. On low-resource languages like Polish, our WER is less than half of the monolingual baseline and even beats the supervised transfer learning method which uses external supervision.”
The restructuring brought in through the JUST framework for multilingual speech recognition includes:
-
A uniform multilingual ASR system that jointly optimized the unsupervised contrastive loss and MLM loss together with RNN-T loss.
-
Joint Unsupervised and Supervised Training framework for multilingual ASR performance is validated on a public multilingual ASR dataset, MLS.
-
Significantly outperforms the monolingual baselines, a SOTA 2-stage pre-train-finetune model XLSR.
Moving forward in the end-to-end approach for multilingual ASR, it would be interesting to add complex languages like Mandarin. For more details on the methodology of the research, check out the open-sourced research paper.