In-Memory Search and Compute: The “In” Thing for Flash
May 15, 2023
Blog
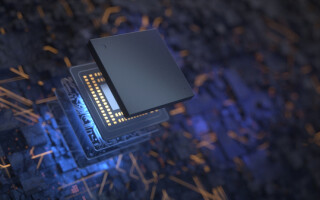
Advancements in artificial intelligence (AI), Internet of Things (IoT) and 5G communications have ushered in an era of unprecedented data generation and traffic. Processors move and crunch data as quickly and efficiently as their architectures allow.
Emerging applications implementing AI have an insatiable appetite for performance that strain traditional solutions trying to keep up with market expectations. Emerging solutions for higher-performance data recognition are starting to resemble the same architectural changes that happened with network interface controllers (NICs) to create “smart” NICs, as one example. Recently, we’ve seen the emergence of compute-in-memory (CIM) or in-memory-search (IMS) solutions for far memory, a technique whereby memory management is offloaded from host processors to the memory devices themselves to reduce bus traffic and increase performance.
AI spans the spectrum in where and how it’s applied – and its ecosystem is extensive: cloud computing and storage “hovering” over arrays of servers connected to a myriad of edge devices targeting applications such as consumer, automotive, industrial, medical, communications, and e-commerce. Each of those edge devices requires advanced architectures and powerful processors, memories and sensors. What all those layers have in common are requirements for real-time data processing, high bandwidths, low power consumption, and system cost efficiency.
To meet the demands of effective AI operation, efficient memory architectures are emerging, such as Compute Express Link (CXL), to support a heterogeneous memory landscape, distributing the workload across these memories. For example, new memory-centric computing is fast-emerging as a technology to scale traditional processor-centric computing. It is achieved by either minimizing data movement, or the negative effects of it, reducing energy and processing time. For example, new flash memories are being developed for nonvolatile data and code storage, which features high-density, low-power, and small-form factors, this type of memory is a logical choice for accelerating AI computation.
Flash memory is poised to take on a greater role in such AI applications by relieving processors of some of their compute tasks, thus freeing them up to provide critical parallel processing operations. Whereas traditionally flash memory would serve primarily as a storage device, within a system relying on a larger percentage of DRAM, the new memory-centric-computing paradigm features flash memory that can process some tasks that had been relegated to the host processor; thus, reducing the amount of required DRAM. Interweaving flash memory and processing resources improves data bus movement, resulting in significantly reduced bill-of-materials costs, lower power, and increased system performance.
With AI applications, its enormous data volume traditionally requires processing resources to both perform core compute functions and load/store functions for data from memory. Shifting some of the data processing responsibility to flash storage, as IMS is intended to do, significantly reduces the energy that would have been consumed in a much larger set of memory operations.
Where best to use this type of IMS? AI-demanding applications include fingerprint, facial, or voice recognition, large data searches, DNA matching, and general pattern recognition.
We at Macronix aren’t just sketching out this concept on a whiteboard; we’re already making CIM and IMS a reality, through our FortiX flash memory platform. Based on our 3D NOR (32 layers) and 3D NAND (96 layers) flash devices, FortiX builds pattern recognition search functions into the memory; it’s high-bandwidth internal-search function maximizes I/O for optimum data acceleration, lowers power consumption and increases system performance.