Shrinking Automotive Power Chips to Advance Automotive AI
November 21, 2024
Blog
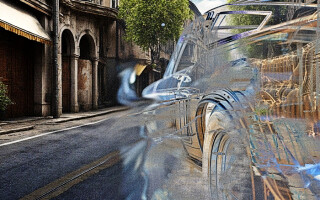
The much-lauded potential of artificial intelligence certainly extends across the automotive industry, with uses ranging from vehicle design and manufacturing to actual in-vehicle applications like safety and infotainment systems.
AI is perhaps most commonly discussed in the context of large language models (LLMs) like ChatGPT, Gemini and Claude, which utilize a natural language interface to search a massive database of responses and then provide the response it deems to be most topical. LLMs are also helpful in a car.
One example: today, in-vehicle voice commands are often haphazard—the user must use certain phrases or reference specific applications so that the computer in the car can ‘understand’ them. Essentially, a very unnatural version of speech.
AI running locally in the vehicle can solve that problem. Volkswagen presented the integration of ChatGPT into its IDA voice assistant at CES 2024, recently launching the service in the U.S.. Other car companies are addressing accessibility, using LLMs that function multilingually.
But behind the AI technology that adjusts a vehicle’s temperature when you tell your voice assistant you are feeling hot or cold, or behind the AI technology that allows voice commands to function regardless of the speaker’s language—to name just two use cases—lies an enormous amount of data to process; and, thus, an enormous amount of power to consume during its processing.
The term AI data center has quickly become ubiquitous to describe the advanced facilities needed to meet the growing electricity, cooling and processing demands of broader-scale AI workloads. While smaller in scale and much more localized, in-vehicle AI deployments consume an enormous amount of energy—and generate a significant amount of heat—at the individual vehicle level.
At the vehicle level, the data consumption strain presents a very real issue, with several upgrades, like autonomy and advanced driver assistance system’s accelerating bandwidth demand in both commercial and passenger vehicles. AI is another element straining this demand; for example, the implementation of LLMs in vehicles to upgrade, for example, voice command requires significantly more efficient processors, larger data storage capabilities and faster data movement.
Just as semiconductors based on smaller geometries are easing the demands of AI in data centers, smaller automotive semiconductor geometries will play an outsized role in determining whether commercial and passenger vehicles can keep up with the commensurate heat and data processing increase.
For automotive products, the 7nm process is leading edge, and automotive chips are now entering the market at this geometry. However, achieving that geometry has faced significant challenges: how do you reduce chip size—and thus decrease power dissipation—while maintaining or increasing network speed, which is only becoming more vital as vehicles continue to evolve, including with AI?
Plus, the automotive process requires additional measures to ensure both high quality and OEM lifetimes of up time compared to regular data center parts. Advanced-geometry processes are the only way to reduce the power dissipation baseline. But shrinking geometries pose additional problems beyond power dissipation; for example, the implementation of analogue and mixed signal electronics—necessary for networks—becomes ever harder.
Developers will create AI applications for vehicles faster than advanced hardware can be conceptualized, developed, tested, mass-manufactured and implemented. So, automotive hardware engineers today face an added challenge of future-proofing in-vehicle networks for ever-growing AI power demand—on top of the growing power demand of more advanced driver assistance systems, greater autonomy and more software-defined features.
The road ahead for in-vehicle automotive AI is undoubtedly exciting; ultimately, advanced automotive semiconductors at 7 nm and beyond will determine the optimization of those features.