The Great Compute Migration: From Cloud Computing to Edge Supercomputing
April 09, 2021
Story
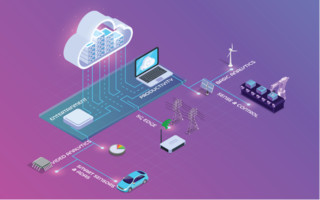
When people and devices are confronted by a tsunami of data where every millisecond counts, edge supercomputing is the real-time processing solution for today’s increasingly connected world.
Recent advancements in computing performance, software algorithms, connectivity, and deep learning are revolutionizing human-machine interaction. By applying these innovations to consumer products, for example, mobile devices can deliver a more powerful user experience. In transportation, vehicles can encapsulate smart features that make them safer and more efficient. Unmanned aerial vehicles (UAVs), or drones, can enable safety inspections of remote pipelines and infrastructure assets to be accomplished without putting humans at risk. In industrial applications, developers can achieve greater levels of efficiency, precision and scalability of manufacturing processes with highly intelligent robotics. Consumers also can unlock the benefits of the Internet of things (IoT) and smart home automation, freeing up time to do more of the things we enjoy.
The proliferation of sensors and cameras in today’s IoT applications, autonomous vehicles, and industrial robotics calls for new, high-performance edge processing solutions that improve computational power while consuming less energy and enhancing security and privacy. Although cloud computing has revolutionized how we process and store large data sets, several handicaps, such as performance and bandwidth, limit autonomous applications as edge-based decisions must be made with minimal latency.
With the explosion of IoT technology and sensors in recent years, there is no easy way to manage and leverage all the data generated continuously by billions of connected devices. Realizing the promise of artificial intelligence (AI) requires access to huge amounts of sensor data for virtually instantaneous decision making. Moreover, direct communication among sensors and compute resources is essential for real-time decisions. These new demands are driving the industry toward edge supercomputing, which enables data acquisition and processing to occur at the edge of the access network and much closer to end users.
Managing the Data Deluge
Consider the vast installed base of sensor-laden IoT devices generating a deluge of data. According to Verizon, there are more than one million connected devices per square kilometer. These IoT devices are ubiquitous and growing in numbers. From security cameras in our homes and offices, to personal medical devices and agricultural sensors, to the smartphones we carry everywhere. Verizon estimates that a single connected car generates more data than all of Facebook on any given day. Multiply that level of data output by all of today’s connected devices, wireless sensors, and robots deployed worldwide, it’s easy to see we are facing a tsunami of data that can swamp our ability to make real-time decisions.
Unfortunately, an estimated 80% of edge data goes to waste because it cannot be transmitted to the cloud for processing due to bandwidth, latency, privacy, or cost constraints. To deliver on the promise of AI and autonomy, we must radically improve networking and computational efficiencies. This includes the ability to learn continuously on the edge as opposed to relying on dizzying amounts of data uploads to the cloud to perform fully centralized training of deep neural networks.
Existing networking and cloud computing technologies are not optimized to handle the flood of edge data generated by IoT devices. High-performance, power-hungry servers used in hyperscale data centers are unwieldy and too costly to deploy close to the edge. System and network architects have envisioned solutions to this data challenge: add more computational intelligence to the edge instead of the cloud. As this trend solidifies and expands, new growth in computing infrastructure will arise much closer to end users at the network edge, outside the data center domain.
According to Forrester Research, the following factors are driving growth in edge computing:
-
Ongoing expansion of the IoT and machine-to-machine (M2M) connectivity
-
Sophisticated algorithms and new applications, such as AI, machine learning, neural networks, autonomous vehicles and virtual/augmented reality, all requiring low latency and high reliability
-
Bandwidth and connectivity limitations impacting cloud computing
-
The rising cost of data storage and transmission
-
An increasingly distributed and mobile workforce
-
New and emerging data privacy concerns and requirements.
The Rise of Edge Supercomputing
In this decade and beyond, we will see innovations in high-performance computing outside the data center built on the back of edge computing and edge server technologies. And we will see the rapid rise of a new compute paradigm: edge supercomputing.
The following figure shows the tradeoffs of computing infrastructure characteristics as we move away from data center models and closer to intelligent, computationally powerful edge devices.
As intelligent edge devices continue to proliferate in the field, the investment and time to market required to embed high-performance compute capabilities into these devices will only accelerate. Real-time applications such as autonomous vehicles and industrial IoT equipment will require significant onboard computing resources. Bandwidth-constrained applications also can be addressed more efficiently by adding on-premises servers or edge data centers.
A Shift in Strategies and Architectures
Because machine intelligence at the edge relies on various sensors embedded in devices that make real-time decisions, the computational power and low latency required are greater than that which current data processing infrastructure (i.e., the cloud) is equipped to handle on a massive scale. These emerging requirements are creating a shift in how and where data is processed.
Many data centers are moving portions of their computing resources closer to the devices receiving and sending data. More users of AI-enabled devices are choosing to process data on-site rather than in the cloud. With data stored and processed locally rather than transmitted to the cloud, edge computing enhances many aspects of security and privacy. Edge computing also opens up new opportunities for innovation to meet the growing demand for high-performance, low-latency, energy-efficient IoT products and smart, autonomous applications.
The ongoing shift toward edge computing will require reimagined IT strategies and architectures. The following factors are important considerations for the new edge supercomputing paradigm:
-
Realign support operations to the edge - Extend software support beyond x86 CPUs and compute unified device architecture (CUDA) GPUs to new architectures optimized for edge or embedded servers. Deploy flexible hardware architectures to run different types of workloads in multi-tenant environments leveraging evolving algorithm workloads.
-
Extend dev-ops - Expand dev-ops beyond the cloud to edge devices and everywhere in between.
-
Reprioritize capital allocation - Explore investments in deploying on-premises edge servers and/or increasing edge data center capacity.
Adding high-performance edge processing capabilities to today’s operational architectures is as critical to IoT and AI infrastructure as expanding cloud compute capabilities was in the past decade. Despite progress in many areas of edge processing, developers deploying advanced algorithms on the edge remain resource constrained. The full potential of edge-based machine intelligence to improve tasks and processes has not been achieved.
Developers must tailor AI and high-performance workloads for optimized target hardware rather than the other way around. Hardware should be purpose-built for these demanding edge workloads. Developers seeking to create algorithms for new application challenges require room for experimentation and innovation. Currently available edge computing products may enable design flexibility, but they lack the processing power to turn ideas into market-viable applications that can be deployed on a large scale. The solution is edge supercomputing – an entirely new hardware and software architecture that combines high-performance computing with sophisticated AI capabilities.
The benefits of deploying edge supercomputing across multiple applications and markets will be transformative for people, workplaces, industries and cities everywhere. As real-time decision making for intelligent edge devices becomes a reality, we will experience a world of possibilities we’ve yet to imagine and untold innovations that will make our lives safer, more secure, and more productive.