Xilinx Zync stack enables machine-learning applications
March 21, 2017
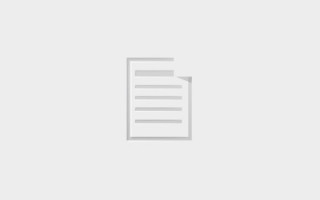
Our readers, who I believe are a great representation of the embedded community, have told us that they really like the Xilinx Zynq programmable processor architecture. It’s useful in a wide array...
Our readers, who I believe are a great representation of the embedded community, have told us that they really like the Xilinx Zynq programmable processor architecture. It’s useful in a wide array of applications, particularly those in the IoT and Industrial IoT spaces. So, I was a little surprised when I visited with the Xilinx folks and they explained how they were going all in on vision applications.
Not that vision isn’t very important, but it is limiting. After hearing the complete story, I’m somewhat a believer (and if you know me, “somewhat of a believer” is generally the best you get). When I say “vision,” that includes machine-learning applications as well, and that’s becoming very important in our space.
To that end, the company announced last week at Embedded World its reVision stack. The stack enables a broader set of developers, even those with limited hardware design experience to develop intelligent vision guided systems more easily, combining machine learning, computer vision, sensor fusion, and connectivity.
Potential applications include high-end consumer, automotive, industrial, medical, and aerospace/defense, as well as drones and autonomous vehicles. They also include a term I recently learned, co-bots, which is short for collaborative robots. These are robots that work alongside humans. This is actually harder than it sounds and there are some pretty stringent standards you have to adhere to when implementing this technology.
Accoding to Xilinx, reVision enables up to 6X better images/s/W in machine learning inference, 40X better in computer vision processing, and 20% the latency over competing embedded GPUs and typical SoCs. The stack, available in the second quarter of this year, includes support for the most popular neural networks including AlexNet, GoogLeNet, SqueezeNet, SSD, and FCN.