Why Organizations Should Consider Predictive Maintenance Models
June 14, 2021
Blog
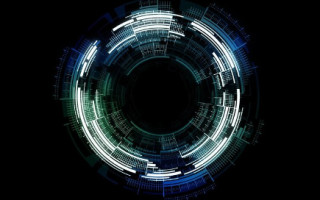
For industrial equipment operators, who are constantly being pushed to reduce downtime (leading to more frequent equipment use) and decrease maintenance costs (which typically rise as equipment is used), predictive maintenance has shifted from a “nice-to-have” to a must-have.
Once implemented, an effective predictive maintenance system is capable of anticipating and identifying potential equipment malfunctions, enabling operators to preemptively schedule repairs, prevent catastrophic system failures, and minimize disruption to a factory’s operations — ultimately benefitting a company’s bottom line.
Skepticism of predictive maintenance’s measurable benefits still exists with executives often mislabeling predictive maintenance as a “black box” solution. They picture an application being fed a machine’s operational data and somehow predicting its remaining lifespan. To the extent executives understand the process, they cannot imagine how an algorithm could have enough equipment failure data to be functional.
Realistically, this “black box” stereotype is not only inaccurate, but ignores the role played by domain knowledge, or expertise unique to the solution’s target environment. To cite one example, the industrial engineers who develop, monitor, and maintain a factory’s operations play a pivotal role in developing the algorithms that predict and detect their equipment’s potential failure.
How Software Bridges the Data/Engineering Knowledge Gap
Predictive maintenance is generally led by data scientists with mathematics backgrounds, who can sometimes lack expert knowledge of the domain their algorithms are supporting. Therefore, the value that engineers bring to the table is their domain knowledge, making them key in developing effective algorithms for predictive maintenance. Software plays the role of bridging the gap between these data scientists and engineers.
Yes, companies need to know what failure data looks like when training these algorithms. That type of data is typically unavailable given that equipment rarely breaks down, and it’s not financially viable to run equipment to intentionally fail for the sole purpose of collecting data. That is where simulation software such as MATLAB comes in as it makes it easier both for engineers to feed data related to their area of expertise into a predictive model, and for data scientists to use that data to train the models.
Users who lack familiarity with data science can still use the software to implement different data processing techniques, identify potential problems, and test potential solutions. Users unfamiliar with predictive maintenance can also use it to generate simulated failure data, strengthening the algorithm’s predictive maintenance capabilities and ensuring less real-world data is needed for the algorithm to be effectively trained.
Oil field service company Baker Hughes, for example, used MATLAB to develop pump health monitoring software, reducing equipment downtime costs by as much as 40 percent and cutting down the onsite need for additional trucks. Meanwhile, packaging and paper goods manufacturer Mondi was able to use MATLAB to develop a predictive maintenance application capable of identifying potential equipment issues in a matter of months.
What’s Next for Predictive Maintenance in 2021?
Most predictive maintenance algorithms are currently located in the industrial spaces and facilities using them – preferably close to equipment, like an edge server that collects data from a local power generator, production facility, or extraction equipment. While this can provide a solid foundation for getting started with predictive maintenance, organizations should also consider cloud solutions in addition to on-premises. Hosting in the cloud can be beneficial for some cutting-edge algorithms that need powerful processors to produce their insights like artificial intelligence (AI)-powered software.
Consequently, in addition to an increased number of industrial controllers and edge computing devices, companies should expect to see more competitors adopting cloud systems, in order to optimize the functionality of their production software. While some manufacturers have expressed skepticism of the cloud due to factors such as data ownership and security, they should nevertheless prepare for the reality of cloud-based predictive maintenance. As the cloud is capable of collecting data from multiple sources, it is thereby training predictive maintenance algorithms more efficiently.
Of course, predictive maintenance is only one measurable benefit of many that businesses incorporating AI into their production lines stand to reap. The organizations that ignore AI are increasingly at a competitive disadvantage and will continue to remain that way unless they explore AI integration in some way. The good news is that AI and its benefits, including predictive maintenance, are within the reach of every company, and there are more than enough resources about the subject to help them learn.
Philipp Wallner is the Industry Manager at MathWorks, where he works closely with the company’s worldwide offices to sharpen MathWorks' strategy and offerings in Digital Transformation, AI, and Industry 4.0.