Google Presents Predicting Text Readability Through Reader Interactions
January 10, 2022
Blog
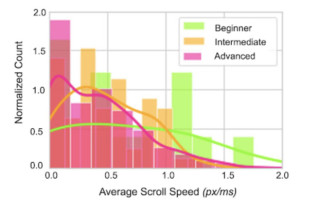
Prediction of text readability has become an area of research due to the increasing complexity in the educational content.
With the onset of the pandemic, there has been an exponential rise in the number of children falling short of the minimum proficiency level of reading. This has boosted research for predicting the readability of text as reading on devices has taken place of the traditional forms. The potential to evaluate the reading interactions as to how the reader scrolls through the text can give insight on the level of understanding when reading a particular text. Such parametric evaluation is important for designing educational applications for low-proficiency readers and new language learners.
Google’s presentation at CoNLL 2021 provides the findings of 518 participants to investigate the relation between scrolling behavior and readability of text. Through open-source datasets, the research demonstrates that there are substantial differences as to how readers interact with text depending on the text level. These measures can be employed to predict text readability, background of the reader that impacts the reading interaction and factors adding to the text difficulty.
[Image Credit: Google AI Blog]
One of the most challenging aspects of the research was to analyze which scrolling behaviors were most impacted by the text complexity. Using linear mixed effect models on multiple measure points of each participants reading more than one text and multiple participants reading the same text. The model concluded that the differences in reader interactions depended on text complexity, leaving out other random effects. When added speed, acceleration and regressions as inputs to the machine learning algorithm, the support vector machine predicts if the text is advanced or elementary based on the scrolling patterns of the readers. The metric to analyze the accuracy of the model was done using f-score with 1.0 reflecting the perfect classification accuracy. The initial results with f-score on predicting the readability using interaction features was 0.77 (77%).
To improve the readability models, more interaction features were included which improved the f-score of this model from 0.84 to 0.88. In addition to this, the model could significantly outperform the system by employing vocabulary features which increased the f-score to 0.96. But it was important to actually ask the participants the level of understanding on what they had read. The interaction features of the scrolling behavior represented as a high dimensional vector and the data was plotted of each participant using t-distributed stochastic neighbor embeddings. t-SNE is a statistical method for visualizing high-dimensional data. The plot shows the t-SNE projection of scrolling interaction in two dimensions. The color on the plot signified the comprehension score which can be evaluated. The results revealed that the clusters in the comprehension score indicate the correlations between the reading behavior and comprehension.
[Image Credit: Google AI Blog]
After the statistical models, the research extends to understand the reading interactions among various audiences. The comparison was done between the average scrolling speed and the reader’s first language. It can be clearly seen that the speed distribution has changed with the proficiency and first language of the audience. This concludes that the first language and proficiency can affect the reading behaviors of audiences. The lines on the histogram show the trend for each group. The higher average scroll speed indicates that the faster reading time, which means a complex text corresponds to slower scroll speed by advanced readers, is associated with higher scroll speeds by beginners as they interact with the text superficially.
[Image Credit: Google AI Blog]
This marks the first research showing the reading interactions (scrolling behavior) can be used for predicting the text readability.
For more details on the methodology of the research, checkout the open-sourced research paper.