Miniature Imaging System with Edge Intelligence for Remote AI Applications
May 19, 2022
Blog
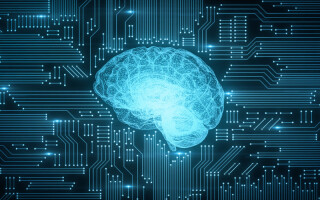
Remote edge AI applications are dependent on the size of the embedded hardware device that carry all the computational power and capabilities for processing edge data at the source. Large, embedded AI accelerators face several challenges in space-constrained systems such as being obtrusive and covert.
In the article titled, “Millimeter-Scale Ultra-Low-Power Imaging System for Intelligent Edge Monitoring,” the researchers developed a miniature millimeter scale imaging system with deep learning and image processing capabilities for edge applications, especially for home-surveillance scenarios. The system uses vertically stacked ultra-low-power ICs and employed dynamic behavior-specific power management, hierarchical event detection, and a combination of data compression methods.
For mm-scale systems, the design approach faces technical challenges such as designing highly area-constrained systems, limitations in energy budget and peak power, limited data storage, costly wireless communications, non-idealities from mm-scale lens, and ultra-low-power front-end.
Starting off with the mm-scale size system, the design requires integration of ultra-low-power ICs and assembling them to reduce the footprint. To power the mm-scale system, the design unit demands a small-sized battery to support the power requirements. Due to the space-constrained system, the imaging system will have limited amount of on-chip and off-chip memory available which limits the storage of image data and complexity of the algorithms.
To address these technical difficulties in designing the mm-scale imaging system, the team took references from the existing work done on low-power image sensors, ultra-low-power processors and mixed-signal vision integrated circuits, low-power wireless communication, efficient neural network accelerator, and optimizing machine learning algorithms for edge computing applications.
Limitations in existing research includes the exclusion of edge computing or not meeting the area and power demands. The research paper proposes the fully integrated mm-scale imaging system as being “the first of its kind.”
Figure: Imager system cross-section
A novel mm-scale imaging system with edge intelligence
Measuring just 6.7×7×5mm size and 460mg in weight, the system uses 150μm ultra-low-power integrated circuits that are designed to be vertically stacked. The method of vertical stacking allows for more integrated circuits to be interconnected within a smaller footprint. This is in comparison to the traditional planar 2D chip-to-chip connection.
The system consists of a base layer integrating several functional hardware devices onto a single IC die, such as master controller, power management unit, and radio IC. At the heart of the mm-scale imaging system is the Arm Cortex-M0 processor core with 16kB SRAM. The power management unit generates several voltage domains from a single battery source to maintain high conversion efficiency under loads.
The next important layer is the ultra-low-power image sensor and image signal processing. The image sensor layer supports motion-triggered 12-bit VGA image capture and near-pixel motion detection on a sub-sampled pixel frame at a maximum rate of 170 frames per second, while the image signal processing performs on-the-fly JPEG (de)compression, optical-black pixel calibration, de-Bayering, RGB-to-YUV conversion, and scene change detection. The neural engine offers 1.5 TOPs/W performance enabling deep neural network-based frame analysis. The other layer includes, ultra-low-leakage flash memory, energy harvester, solar cell, rechargeable lithium battery, and polytetrafluoroethylene (PTFE) tube.
The system integration used two 4-layer 10×10×0.8mm PCBs of which the front side is used for wire-bonding, whereas the passive components and the solar cell layer are placed on the back. Code development for such mm-scale systems is challenging so the team included castellated vias on the outer rims to expose the internal signals.
Figure: (Left to right) Stacked ICs, Encapsulated system under test, Back side of the PCB, standalone mm-scale imaging system
Modifications to the mm-scale imaging system
Power management is the key element for the energy minimization technique due to the huge power consumption by the always-on image sensor performing DNN-based analysis. The process demands for both computation energy and megabytes of DNN parameters stored on- and off-chip.
The dynamic energy-efficient modes such as flash memory ICs are set to sleep mode during motion monitoring, image capturing, and DNN-based scene analysis to only consume 0.003μW. The power management unit is adjusted to each node to maximize the efficiency of the dynamic load by modifying current draw, frequency control, and up/down conversion ratio. On the other hand, hierarchical event detection (HED) algorithm is used to prune out irrelevant events that would otherwise consume energy, particularly when offloading data without determining its value to the application.
Combining weight pruning, non-uniform quantization, Huffman encoding of quantized weights for convolutional layers, and several other methods, the compression of up to 1.5bit/weight is achieved for DNNs. For image compression, the combination of JPEG and H.264 compression methods are used to reduce the data footprint and minimize on the wireless transmission cost.
The H.264 intra-frame compression engine reduces the memory footprint of a VGA frame by 23x. However, through H.264 compression, the system demands for 138% more processing energy than JPEG. Other approaches that are used are change detection engine to achieve 135x compression compared to a VGA frame, and off-system image reconstruction for reducing wireless data transmission. For image correction on mm-scale lens and ultra-low-power front ends, the team proposes image-correcting layers that can be executed on the ISP neural engine (NE) using instructions such as matrix multiplication and convolution.
Conclusion
The paper proposed a novel mm-scale imaging system integrating edge intelligence for remote IoT and edge applications with an average power consumption of 49.6μW and expected lifetime of 7 days without recharging. To achieve the small formfactor, the system uses vertical stacking of the ultra-low-power ICs and manages memory and energy budget constraints through data and energy management methodologies. “Having demonstrated a tiny-IoT intelligent imaging system, analysis through a sociotechnical and ethical lens is an essential next step; we invite future work on topics such as security and privacy,” the team concludes.
More details are available in the research work published on Cornell University’s research sharing platform, arXiv under open access terms.
References
[1] Andrea Bejarano-Carbo, Hyochan An, Kyojin Choo, Shiyu Liu, Dennis Sylvester, David Blaauw, and Hun-Seok Kim. 2022. Millimeter-Scale UltraLow-Power Imaging System for Intelligent Edge Monitoring. In Proceedings of tinyML Research Symposium (tinyML Research Symposium’22). ACM, New York, NY, USA, 7 pages.