AI Will Mature to Solve Real Operational Problems in 2022
February 11, 2022
Story
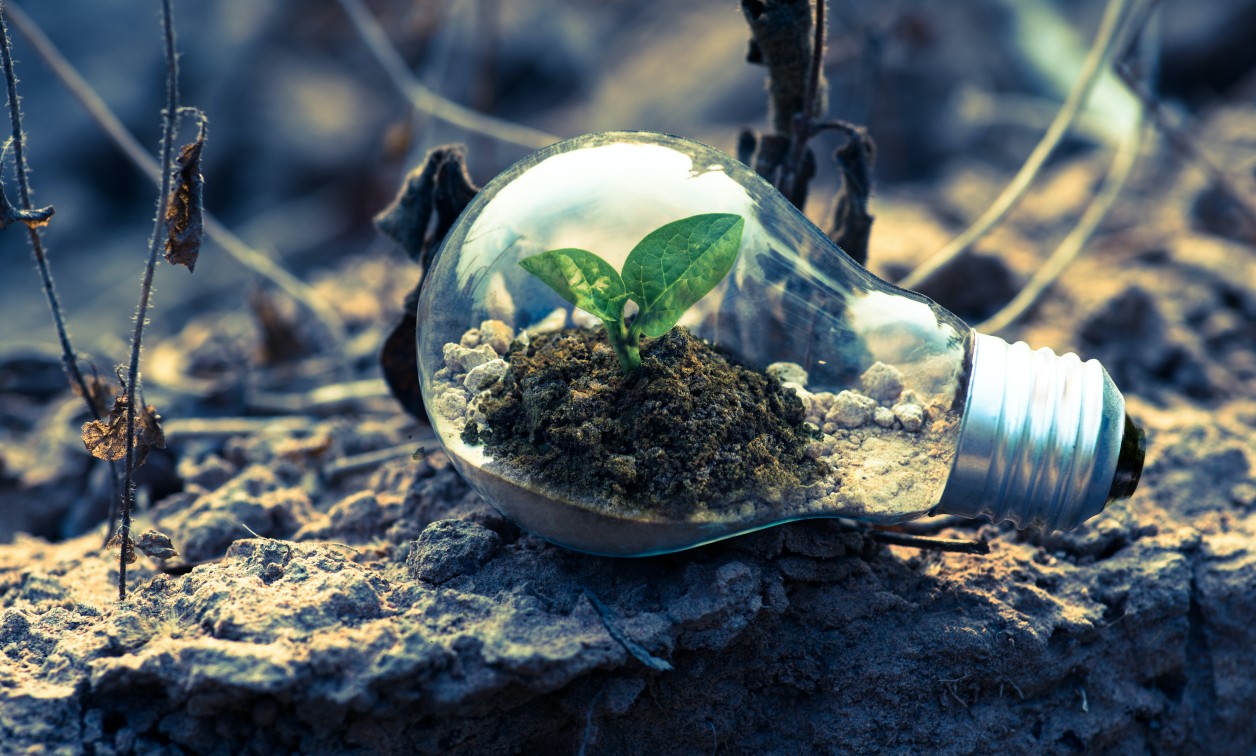
A decade ago AI and ML were considered mysterious and academic, with applicability to real world problems being challenging. The average person would read about something exciting in the press that Stanford or MIT was developing or some experimental software that Google was creating in secret, but it still seemed like science fiction.
To business, ten years ago, it may have meant even less—it represented some new technology that was unpragmatic at best, with no contemporary application.
Fast forward ten years.
The internet, mobile technology, cloud computing: All of these innovations went through the same standard S curve of adoption. Innovators created the new technology and early adopters worked through its initial stages, helping iron out the kinks while figuring out its practical uses. They were followed by the early majority, who streamlined and perfected the technology’s use. Then the late majority adopted it at which point it is hard to remember what prior life was like.
AI and ML's Place on the S Curve
AI and ML are following this familiar path. The implications of what AI and ML can do have become apparent to early adopters. When I hit the road, talking to manufacturers just five years ago, most weren’t familiar with how neural networks, for example, worked – they had heard of them at all. As we progress into 2022 and beyond, there is clear evidence that technologies are moving into the early majority phase as more enterprises ask what AI and ML can do for them.
Here are a few indications: The basic language of AI is more frequently used and better understood in both business and society.
Once the basic language for new technology is understood, it shortens the time for new adopters as the concepts are not foreign to them. Remember when someone first told you to “connect to Wi-Fi” using a “hotspot?” Or when you heard about “conditional formatting” in an Excel spreadsheet? Now those terms are likely part of your daily lexicon.
The same shift is happening in AI and ML — as people learn and get comfortable with the terminology (and stop worrying about looking like an idiot for using terms wrong), they’ll talk about it more. As a result, new adopters will focus less on the tech itself and conceptualize more about its possibility. I am now asked the pragmatic (and, honestly, more pertinent) questions of what problems AI and ML can solve.
From the perspective of business, it means questions like:
- “Could we use AI or ML to reduce our customer wait times?”
- “Could we use this tech to reduce our shipping costs and times, and increase fuel efficiencies?”
- “Could AI or ML help us recognize variations in production that may be preventable and save us time and money?”
- “What is the quality of your action recognition?” Important because defect rates in the best manufacturers are very low, in the 0.001% range and AI is often challenged to be accurate. (Answer: A recent bake off by one of our customers showed that we didn’t miss any of the errors that they threw at our system!)
- “How quickly can we retrain your system when changes occur on the plant floor?” (Answer: in days!)
AI is Moving Out of the Lab and into Operations
While businesses are adopting AI in their operations, they are doing so with the knowledge that access and infrastructure will need to change. They recognize that there will be a paradigm shift in how operations are organized once ML is introduced at scale.
With this shift, everyone from senior leadership to the line associates will see real changes that our customers are already reporting: fewer hours will be spent on data collection. Monitoring and variance analysis will become a task for AI, removing the limitations of human bandwidth and attention to detail. The result will save countless hours that can, instead, be invested in the problem solving process.
In order to see the full potential of AI and ML operations, businesses will have to have a shift in philosophy and ultimately change their relationship with data.
As businesses grow, they implement management systems for monitoring key performance indicators (KPIs) throughout their operations, along with the business processes that deliver the results they are looking for. The larger an operation is, the more critical these systems are and scalability becomes a factor, further emphasizing the need for systems to be standardized and comprehensive.
For example, consider the most famous efficiency management philosophy, the Toyota Production System (TPS). TPS introduced the concept of the “TPS House” which visualizes its tenets for comprehensive management in the structure of a house with pillars. If any one of the pillars is compromised, the whole house potentially suffers. TPS has arguably influenced how every major manufacturer operates.
The adoption of AI and ML in business presents enough of an impact on the flow of information that ML operations must be incorporated into the infrastructure in a similar way as the TPS philosophies. Incorporation may require a new ML house be created and implemented to streamline the technology's efficacy. At the very least, it will become a standard pillar of the next iteration of the House of Lean.
The Global Perspective
As the developed world moves along the curve of adoption for AI and ML, barriers will break down and inevitably the technology will enter into emerging markets. Already, inquiries from BRIC and other emerging countries show that AI is no longer for developed countries only.
Historically, these developing markets have adopted the last several rounds of technology faster than their developed counterparts. Consider the adoption of mobile technology in undeveloped countries — with the absence of old wired telephone infrastructure present in the developed world, there was no hindrance, psychological or otherwise, that would prevent them from leaping to the latest technological solution once made available. As another example, mobile banking, once introduced, vastly outpaced adoption in developing countries. In fact, it is fair to say that with the significant strategic investment the Chinese government is making, they are aiming to be leaders soon.
If this is any indication, it means that once adopted, emerging markets possess the potential to leapfrog developed countries in their adoption, use, and application of AI and ML.
2022 and Beyond
AI and ML are the next wave that will drastically impact industry. 2022 represents a hotbed of possibilities for these technologies. It is critical that organizations make efforts to prepare for these changes. The wave will hit, whether they are ready for it or not.
Prasad Akella is the founder and chairman of Drishti, which provides AI-powered video analytics for discrete manufacturers to digitize their manual assembly operations