Improving GNSS Performance with "Crowd Sourcing" and Cellular Networks
December 08, 2016
Story
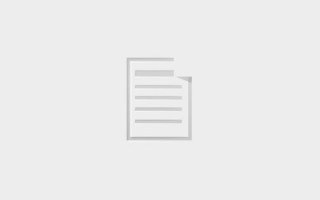
GNSS is becoming one of the most ubiquitous technologies on the planet. As ABI Ressearch puts it, "GPS IC shipments are forecast to reach two billion...
GNSS is becoming one of the most ubiquitous technologies on the planet. As ABI Ressearch puts it, “GPS IC shipments are forecast to reach two billion by 2016 … Along the way, the market will shift from standalone ICs to combo chips and embedded GNSS solutions, with greater emphasis placed on alternative location, MEMS, GLONASS, and other GNSS technologies.”
Today, GNSS is not only found in sat navs and smartphones, but also in a significant portion of the wearable and fitness devices on the market. Further, it is even making the shift from “new-car luxury” to “new-car essential,” as evident in BMW’s decision to ship all new cars with the technology integrated from this month forward.
To obtain a precise location, GNSS positioning systems require signals from four satellites. However, errors can still result from the satellite signal being obstructed, for example by a tunnel or when transiting through an urban canyon. GPS jamming technologies (such as those deployed to obfuscate communications from anti-theft systems or prevent data being transmitted from insurance or telematics boxes) are also a problem, as not only do they block target systems, they also typically create a 500-meter bubble that affects the signals of anything nearby. In the case of emergency vehicles or even low-flying aircraft, the effects can be lethal.
Dead reckoning, where a vehicle’s position can be calculated in three dimensions using additional on-board sensors (such as the vehicle’s odometer and gyroscope), is an effective way to calculate position following the loss of a GNSS signal. This method also has its limitations, however, as in principal dead reckoning technologies have “limited lifetimes” since their positioning calculations are simply probabilities – each mistake in these probabilities can affect subsequent calculations, with the result being an accumulation of errors over time. Therefore, the longer a dead reckoning system runs without a GPS signal, the greater the probability of these errors happening and the less accurate it becomes.
It is also worth noting that not all machine-to-machine (M2M) systems are made to be used on outdoor vehicles. Many are also used to track people and indoor vehicles, for example at an exhibition, an airport, an arena/sports ground, or in a hospital, and here the above limitations also apply. Fortunately, though, there are other ways to calculate position.
Using cellular networks as a backup
For some years now, crowd sourcing (i.e. using an army of consumers to help you generate millions or even billions of data points) has been used to improve data accuracy. Facebook, for example, has its users tag many of the 350 million photos uploaded each day in order to improve its facial-recognition algorithms.
Similarly, another way to calculate position is through observations from a cellular network. As long as there is cellular coverage there will be a specific combination of cells to be continuously monitored and a full set of descriptors that can be reported from a cellular module, including a unique cell ID. However, Mobile operators seldom share this data.
Recently, though, u-blox has undertaken a project to map cell observations in certain regions through an implementation called CellLocate, which incorporates data from GSM, UMTS, CDMA, and, eventually, LTE cellular modules. With CellLocate, cellular modules take unique cell descriptors and combine them with accurate GPS data, then report information on the location of each visible base station back to CellLocate servers. As the technology is “self-learning,” new (anonymous) data points sent from various modules increase the density of cellular observations, thereby making base station coverage maps more accurate.
The cellular modules also benefit from the service as when server descriptors are sent to CellLocate, they receive position estimates in return. The accuracy of reported positions depends on the number of visible cells and the coverage of databases in a specific location, but this can be augmented through complementary third-party databases as well as the “crowd-sourced” observations made by modules in the area.
The service can also outpute information about its own accuracy, as, for example, in vehicular test experiments CellLocate provided position estimates with a typical accuracy of 200 meters (50 percent circular error probable (CEP)) and a limit of 2 km (95 percent probability). The accuracy of the position estimate is dependent upon the environment, usage, and available information, as the value of the uncertainty is greater for vehicles driving in remote areas than for, say, pedestrians moving around a town. As a result, applications can more effectively use position estimates.
As no personal identification data is collected, CellLocate also respects the privacy of its users.
Here is an example of how crowd-sourcing and GNSS can be leveraged in unison to improve location estimates for applications operating in the most difficult circumstances, and drive a range of new products and services in the Internet of Things (IoT).