M.2 Accelerators: AI Inference at the Rugged Edge
July 22, 2022
Blog
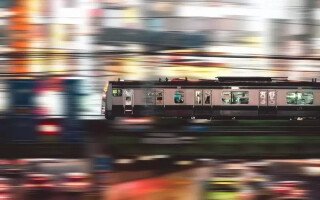
Meeting the hurdles of real-time computing in non-data center environments.
Embedded developers must now consider that processor architectures are struggling to keep up with the increasing demands of deep learning at a time when Moore’s Law is showing signs of wear. At some point, limits prevail, and it is becoming evident that silicon evolution alone cannot support AI algorithms and the orders of magnitude greater processing performance require. Today’s success is directly related to performance acceleration encompassing compute, storage, and connectivity for ideal workload consolidation in close proximity to where data is being generated. It's a necessary balance of performance, cost, and energy that demands a new approach featuring more specialized domain-specific architectures.
System architects now widely believe the only path left for significant improvements in the performance-energy-cost equation is the domain-specific approach integrating M.2 performance acceleration. Domain-specific architectures handle only a few tasks, but they do so exceptionally well – an essential shift for system developers and integrators, as the ultimate goal is to improve the cost versus performance ratio when comparing all accelerators. This includes CPUs, GPUs, and now flexible M.2 module options playing a new role in performance acceleration at the edge.
Getting to know M.2
Developed by Intel® and originally referred to as the Next Generation Form Factor interface, M.2 was designed to provide flexibility and robust performance. Several signal interfaces are supported, including Serial ATA (SATA 3.0), PCI Express (PCIe 3.0 and 4.0), and USB 3.0. M.2 also features a spectrum of bus interfaces, enabling easy adaptability – integrated expansion slots can handle all types of different performance accelerators, storage protocols, I/O expansion modules, and wireless connectivity.
M.2 is compatible with legacy and contemporary storage protocols, supporting both SATA and NVMe (Non-Volatile Memory Express). With its Advanced Host Controller Interface (AHCI), the legacy-standard SATA storage protocol is defined by Intel® as optimizing data manipulation via spinning metal disks in HDD (hard disk drive). NVMe offers an alternate option that instead takes full advantage of NAND chip (flash) storage and the PCI Express Lane to offer lightning-fast solid-state drive (SSD) storage.
Data everywhere, all the time
CPU/GPU-based designs have helped mitigate the effects of a slowdown in Moore’s Law, yet these processor architectures still may struggle with automation and inference applications. The real-time data handling required is particularly challenging at the rugged edge, where systems depart from the rigid controls of a centralized data center structure. Price and performance ratios are at odds, and the overall acceleration strategy must consider compute, storage, and connectivity simultaneously. Balancing these factors is imperative to effective workload consolidation close to data sources, even in increasingly rigorous physical settings.
Using M.2 accelerators to illustrate performance, domain-specific architectures can control inference models 15 to 30 times faster than networks incorporating CPU/GPU technology. At the same time, energy efficiency improves 30 to 80 times. General-purpose GPUs can certainly deliver the massive processing power required to handle advanced AI algorithms; however, they are becoming more and more unsuitable for edge deployments, especially those characterized by remote or unstable conditions. The upfront cost of the GPU itself, along with power consumption, system footprint, and thermal management challenges may create even greater operating costs. In contrast, M.2 acceleration modules and other specialized accelerators such as TPUs from Google are power-efficient and compact. These components are specially designed to run machine learning algorithms with advanced performance at the edge, but within a reduced form factor and power budget.
Keeping in step with evolving data needs
Cognitive machine intelligence is driving advances in all kinds of applications – from within passenger vehicles, to the factory floor, to global infrastructure environments like ports, airports, and train stations. Because these diverse real-world environments have very different needs, processing automated or AI tasks at the data center level is typically inefficient and costly. At the same time, they are the types of industries and applications seeking to maximize competitive advantage with new data-driven services and capabilities. This is where M.2 accelerators create an advantage, providing an option for data-intensive processing in contrast to data center characteristics.
These are the industrial computing systems that uniquely tap into mechanical and thermal engineering to address environmental issues such as strong vibration, severe temperatures, and the presence of moisture or dirt. Optimized industrial-grade computers are also validated to execute functions with extreme processing power and storage capacity, built to eliminate downtime and ensure stable 24/7 operation. Deployed at the rugged edge, these specialized inference computers must not only endure temperature extremes, but also accommodate questionable power sources and mitigate kinetic factors as they process great volumes of data through a wide variety of I/O ports. Rich wireless connectivity permits uninterrupted communication, monitoring, data transfer, and automation to meet the incredible demands of rugged edge computing.
M.2’s domain-specific approach empowers system architects to design solutions that precisely meet the compute requirements of AI workloads in these types of challenging edge environments. Performance, power, and cost can all be considered and ideally balanced. Integration for AI inference is seamless, resulting in hardware engineered for data-intensive performance directly through the PCIe I/O bus. Advanced computer vision and edge computing applications can process and analyze large volumes of data for rugged edge computing.
Combining next-generation processing and high speed storage technologies with the latest features of IoT connectivity is a new and more holistic strategy for developers. The result is an ideal balance of processing dedicated to inference workloads that enable machine learning at the edge. Inference models can be managed faster and more efficiently via an M.2-based system versus a comparable system relying on CPU/GPU technologies. And with clear differentiation between a general-purpose embedded computer and one designed to handle inferencing algorithms via M.2 acceleration modules, more industries can capitalize on data in real-time for improved services, decision making, and overall competitive value.

Premio's AI Edge Inference Computers are designed from the ground up to deliver holistic inference analysis at the rugged edge. Blending next-generation processing, high speed storage technologies, and advanced features of IoT connectivity options, systems also integrate M.2 performance accelerators to ensure a balance of performance, power, and cost.